Ethical Implications of Using Machine Learning Algorithms in Psychotechnical Assessment Processes
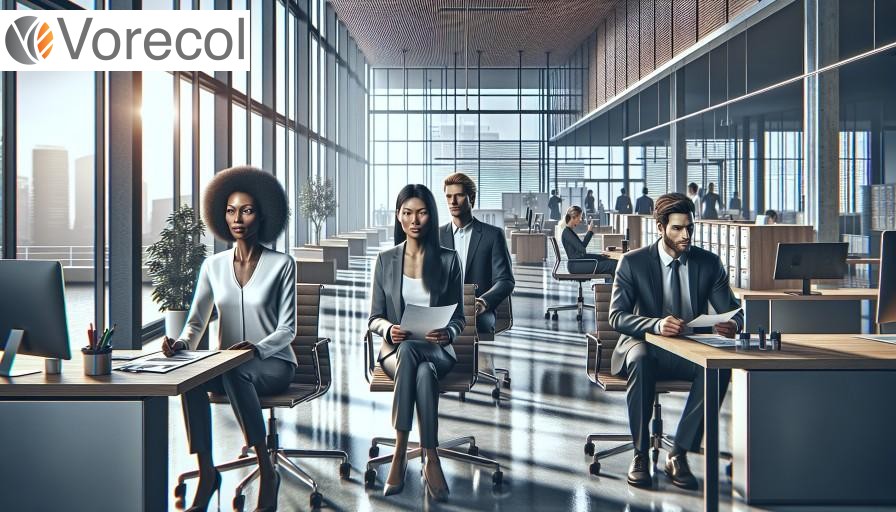
- 1. Understanding Machine Learning in Psychotechnical Assessments
- 2. Potential Biases in Algorithmic Decision-Making
- 3. The Role of Data Privacy in Machine Learning Applications
- 4. Ethical Considerations for Test Fairness and Validity
- 5. Impacts on Employment and Career Opportunities
- 6. Transparency and Explainability in Algorithmic Processes
- 7. Future Directions: Balancing Innovation with Ethical Standards
- Final Conclusions
1. Understanding Machine Learning in Psychotechnical Assessments
In recent years, organizations like Unilever have begun to revolutionize their psychotechnical assessments by integrating machine learning algorithms to enhance their recruitment processes. In 2018, Unilever reported a significant reduction in the time it takes to screen candidates—down from four weeks to just 48 hours—by utilizing AI-driven video interviews that analyze candidates' facial expressions, tone of voice, and language patterns. This transformation not only reduced biases in hiring but also improved the quality of new hires, with a 16% increase in employee retention rates after the implementation of these advanced assessment tools. For companies looking to adopt similar measures, it's essential to focus on the ethical use of data and ensure that algorithms are trained on diverse and representative datasets to avoid perpetuating historical biases.
Another notable example comes from IBM, which has developed a sophisticated machine learning model that evaluates job candidates' cognitive, emotional, and social skills through gamified psychotechnical assessments. These games collect data on how candidates solve problems and interact with virtual environments, providing insights that traditional assessments might overlook. In a pilot study, IBM found that their AI-driven assessments could predict a candidate’s future job performance with an accuracy of up to 87%. Companies facing similar challenges should consider incorporating gamification and multifaceted assessment methods that not only gauge technical skills but also offer a holistic view of candidates’ capabilities, fostering a more dynamic and inclusive hiring process.
2. Potential Biases in Algorithmic Decision-Making
In the world of algorithmic decision-making, the story of the Australian government’s " robo-debt" scandal serves as a stark reminder of potential biases. In this case, an automated system was used to recover welfare payments, but it was built on the flawed assumption that income data provided by recipients was always accurate. As a result, many Australians were falsely accused of owing money, leading to severe financial stress for some and even self-harm in the worst cases. This incident highlights that algorithmic models can reflect the biases of the data they are trained on. To mitigate such risks, organizations must engage in rigorous bias detection and mitigation strategies, including diverse data sets and regular audits of algorithm performance.
Another compelling narrative emerges from the hiring practices of Amazon, which developed an AI recruiting tool to streamline hiring. However, the tool demonstrated bias against women, as it was trained on resumes submitted over a 10-year period, predominantly from male candidates. This revelation forced Amazon to scrap the project, illustrating how even well-intentioned algorithms can perpetuate systemic biases if not carefully monitored. To address similar challenges, businesses should implement a continuous feedback loop with stakeholders, incorporate diverse perspectives during model development, and prioritize transparency in their algorithms to build trust and ensure fairness in their decision-making processes.
3. The Role of Data Privacy in Machine Learning Applications
In a world where data-driven decisions dictate business strategies, the significance of data privacy in machine learning applications cannot be overstated. Consider the story of Equifax, which faced a massive data breach in 2017 affecting approximately 147 million individuals. The fallout was severe—not only did it lead to a significant dip in their market value, but it also raised critical questions about how companies manage sensitive information. Companies leveraging machine learning must prioritize ethical data handling to protect user privacy. Implementing practices such as data anonymization and regular audits can help mitigate risks, ensuring that their machine learning models contribute positively without compromising individual privacy.
On a contrasting note, the European organization, GDPR, has established a framework that underscores the importance of data protection in machine learning. After its implementation, companies like IBM adapted by integrating privacy principles into their AI designs. This proactive approach not only safeguarded consumer trust but also positioned them as leaders in responsible AI development. Organizations addressing similar challenges should consider adopting privacy-by-design principles from the outset. This means embedding privacy measures within the development process rather than as an afterthought, and leveraging techniques like federated learning to allow machine learning while minimizing exposure to personal data. By focusing on transparency and accountability, businesses can harness the power of machine learning while respecting user privacy.
4. Ethical Considerations for Test Fairness and Validity
In 2018, a lawsuit against the standardized testing company ACT highlighted the ethical dilemmas faced in ensuring test fairness and validity. Test takers from underrepresented backgrounds argued that the ACT's methods disadvantaged them, leading to skewed results. The controversy forced the organization to reevaluate its testing processes and consider how socioeconomic factors influenced performance. Learning from this case, organizations can implement accessibility measures, such as providing additional resources and practice tests for marginalized groups. This approach not only enhances the validity of the test but also fosters an inclusive environment that encourages all candidates to perform at their best.
Conversely, the GMAT (Graduate Management Admission Test) has made strides in enhancing test fairness by integrating a more holistic approach to admissions. By allowing business schools to consider multiple factors—such as interviews and essays—alongside test scores, the GMAT has improved the predictive validity of its assessments. Data shows that schools embracing holistic evaluation methods see an increase in diversity among admitted students, with a reported 30% rise in enrollment from various racial and ethnic backgrounds in recent years. Organizations aiming to create fair testing environments should consider diversifying assessment methods and actively soliciting feedback from test-takers to adapt and improve their practices continuously.
5. Impacts on Employment and Career Opportunities
In the heart of Detroit, a city that once epitomized the American auto industry, Ford Motor Company embarked on a bold initiative to revitalise its workforce through the integration of advanced technology. As Ford began to adopt automation and artificial intelligence in their manufacturing processes, they faced an age-old dilemma: would this technological enhancement lead to job losses or create new employment opportunities? Surprisingly, Ford reported that by investing in employee training programs, they not only retained their existing workforce but also created approximately 7,000 new jobs, focusing on roles that required higher skill levels. This pivot to upskilling demonstrates that addressing the impacts of technological advancements on employment is not just about fearing change, but embracing it through education and training.
Across the ocean in Germany, Bosch, a global engineering and technology company, faced a similar challenge as it transitioned to smart manufacturing. By establishing the Bosch Academy, the company offered comprehensive training modules in areas such as Internet of Things (IoT) and robotics, thereby equipping employees with the skills necessary for the digital age. The outcome? Bosch reported a 30% increase in employee satisfaction and a 25% reduction in turnover rates within just a few years. This narrative underscores a crucial lesson for companies facing similar technological shifts: rather than viewing automation as a threat, consider it an opportunity to enhance career prospects through strategic investments in workforce development. Organizations should prioritize ongoing training initiatives and foster a culture of lifelong learning to transform potential disruptions into avenues for growth and innovation.
6. Transparency and Explainability in Algorithmic Processes
In the world of algorithmic decision-making, transparency and explainability are no longer mere buzzwords but essential requirements for organizations striving to maintain public trust. Take the example of IBM's Watson, which faced criticism over its lack of transparency when providing treatment recommendations for cancer patients. While Watson demonstrated impressive diagnostic capabilities, many healthcare professionals were left in the dark about how the algorithm reached its conclusions. This lack of clarity led to decreased confidence among practitioners. To address this, IBM subsequently committed to enhancing the explainability of its AI systems, emphasizing the need for robust and interpretable algorithms that can articulate their decision-making processes. Companies should take note: establishing clear communication around AI functionality can not only alleviate skepticism but also foster a collaborative atmosphere between human expertise and machine learning.
Consider also the case of the fintech company ZestFinance, which encountered challenges when their credit-scoring algorithms were perceived as opaque. By incorporating explainable AI frameworks, ZestFinance successfully illustrated how their algorithms assess creditworthiness, leading to a 40% reduction in loan denials for individuals from historically marginalized communities. This revelation underscores the potential of transparency to create more equitable outcomes in algorithmic processes. Organizations can draw inspiration from ZestFinance's experience by implementing strategies such as regular audits on AI performance, user-friendly visualizations of algorithmic processes, and maintaining an open dialogue with stakeholders. By prioritizing transparency, companies empower users and build a lasting ethos of responsibility in technology deployment.
7. Future Directions: Balancing Innovation with Ethical Standards
When the world’s leading fashion retailer, Zara, made headlines for its rapid turnaround in product design, it also faced criticism for its ethical sourcing practices. Balancing innovation with ethical standards is challenging; however, Zara's parent company, Inditex, took concrete steps to address concerns by launching their sustainability program that aims for 100% organic or recycled cotton, linen, and polyester by 2025. They successfully reduced their greenhouse gas emissions by 50% per garment between 2016 and 2020, showcasing that innovative practices do not have to come at the expense of ethical considerations. Organizations can learn from Zara's journey: ensure transparency in supply chains, incorporate sustainability into core business strategies, and actively engage with communities to foster ethical practices.
Similarly, the tech giant Microsoft faced the dilemma of balancing innovation with ethical AI usage. In 2021, they established the Office of Responsible AI to ensure adherence to ethical principles while continuing to develop cutting-edge technology. This initiative resulted in AI tools that not only protect user privacy but also promote fairness and inclusive design. The company reported a 25% increase in customer trust among users after these enhancements. For businesses navigating similar waters, a blend of proactive ethical oversight and innovation can be crucial. Companies should embed ethics into their innovation strategies by establishing clear guidelines, fostering an inclusive culture for diverse perspectives, and continuously engaging with regulatory frameworks to remain ahead of the curve.
Final Conclusions
In conclusion, the integration of machine learning algorithms in psychotechnical assessment processes presents both promising advancements and complex ethical dilemmas. On one hand, these algorithms can enhance the efficiency, objectivity, and scalability of assessments, potentially leading to more informed decision-making in various fields such as recruitment, education, and mental health. However, the reliance on algorithmic decision-making raises significant concerns regarding privacy, bias, and the dehumanization of evaluation processes. It is imperative for stakeholders to recognize these ethical implications and strive for a balanced approach that prioritizes the well-being and dignity of individuals being assessed.
Furthermore, the deployment of machine learning in psychotechnical assessments necessitates a stringent framework of accountability and transparency. Practitioners must ensure that algorithms are rigorously tested for fairness and accuracy, mitigating the risk of perpetuating existing biases that could adversely affect marginalized populations. Engaging in an open dialogue among technologists, ethicists, and policymakers can foster a collaborative environment that prioritizes ethical standards while embracing the benefits of technological innovation. Ultimately, the ethical use of machine learning in psychotechnical assessments will require ongoing commitment and vigilance to navigate the intricate interplay between technology and human values.
Publication Date: September 15, 2024
Author: Emotint Editorial Team.
Note: This article was generated with the assistance of artificial intelligence, under the supervision and editing of our editorial team.
💡 Would you like to implement this in your company?
With our system you can apply these best practices automatically and professionally.
PsicoSmart - Psychometric Assessments
- ✓ 31 AI-powered psychometric tests
- ✓ Assess 285 competencies + 2500 technical exams
✓ No credit card ✓ 5-minute setup ✓ Support in English
💬 Leave your comment
Your opinion is important to us